In the world of consumer health products (whether supplements, skincare, superfoods, or pet health) real-world evidence (RWE) is becoming a key factor in validating product claims. While traditional clinical trials provide controlled results, RWE data analysis offers a deeper understanding of how products perform in everyday conditions.
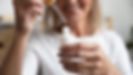
With the right analytical techniques, brands can uncover patterns, validate claims, and optimize marketing strategies. In this blog, we’ll focus on the role of data analysis in real-world evidence, exploring methods, tools, and best practices for extracting actionable insights.
Key Data Analysis Methods in Real-World Evidence
Descriptive Analytics: Understanding Consumer Trends
Descriptive analytics focuses on summarizing historical data to identify patterns. In RWE, this method is used to analyze consumer feedback, product usage trends, and demographic insights.
For example, a supplement brand might use descriptive analytics to determine the most common age group using their product and their primary reasons for purchase. This helps refine target audience strategies.
Predictive Analytics: Forecasting Outcomes and Trends
Predictive analytics leverages machine learning and statistical models to anticipate future trends based on past data.
Example: A skincare company analyzing historical consumer reviews and environmental factors (e.g., humidity levels) might predict how well a moisturizer performs in different climates, allowing them to create region-specific marketing campaigns.
Sentiment Analysis: Extracting Consumer Perception
By applying natural language processing (NLP) to online reviews, surveys, and social media comments, sentiment analysis helps brands measure consumer satisfaction and identify common concerns.
Example: A pet supplement brand could use sentiment analysis to track how customers feel about their product’s effectiveness over time, spotting emerging concerns before they affect sales.
Comparative Analysis: Benchmarking Against Competitors
This method evaluates a product’s performance compared to competitors by analyzing external datasets, including reviews, sales trends, and third-party studies.
Example: A superfood brand could compare its product’s perceived health benefits against similar items in the market, adjusting formulations or marketing messages accordingly.
Cluster Analysis: Identifying Consumer Segments
Cluster analysis groups consumers with similar behaviors, preferences, or characteristics. This is valuable for personalizing marketing efforts and optimizing product recommendations.
Example: A supplement company might discover that one segment of users prioritizes energy-boosting benefits, while another values stress reduction. This insight allows for more tailored advertising.
Tools and Technologies for RWE Data Analysis
AI-Powered Analytics Platforms
AI-driven tools like IBM Watson, Google Cloud AI, and SAS analyze large datasets quickly, identifying trends and correlations that may not be obvious through manual review.
Data Visualization Software
Tools like Tableau and Power BI make it easier to interpret complex datasets through interactive dashboards and reports.
NLP and Text Mining Tools
Google’s Natural Language API, MonkeyLearn, and RapidMiner help brands analyze consumer sentiment across online reviews, social media, and customer support inquiries.
Statistical Software
Programs like R, Python (Pandas, SciPy), and SPSS are commonly used for deep statistical analysis and predictive modeling in RWE studies.
Best Practices for Effective Real-World Evidence Data Analysis
1. Ensure Data Quality and Integrity
Before analysis, brands should clean and preprocess data to remove errors, duplicates, and inconsistencies. High-quality data leads to more reliable insights.
2. Use a Multisource Approach
Combining data from multiple sources—wearable devices, online reviews, consumer surveys, and e-commerce analytics—creates a more comprehensive picture of real-world product performance.
3. Focus on Actionable Insights
Data analysis should go beyond numbers—it should provide clear, actionable takeaways that inform product development, marketing strategies, and consumer engagement.
4. Maintain Data Privacy and Compliance
When analyzing real-world data, brands must adhere to privacy regulations such as GDPR and CCPA. Transparency about data collection and use builds consumer trust.
5. Continuously Monitor and Adapt
Real-world evidence analysis should be an ongoing process. Regular monitoring allows brands to adapt quickly to consumer feedback, emerging trends, and market shifts.
Driving Business Growth with RWE Data Analysis
Real-world evidence data analysis provides brands with invaluable insights into consumer behavior, product performance, and market trends. By leveraging advanced analytics methods, brands can validate claims, personalize marketing, and stay ahead of competitors.
As consumer demand for data-backed claims grows, investing in robust RWE analysis will be essential for building credibility and driving business success.
Is your brand ready to harness the power of real-world data? Explore how we conduct customized clinical trials at Citruslabs.